Top Data Analysis Tools for 2024: A Comprehensive Comparison
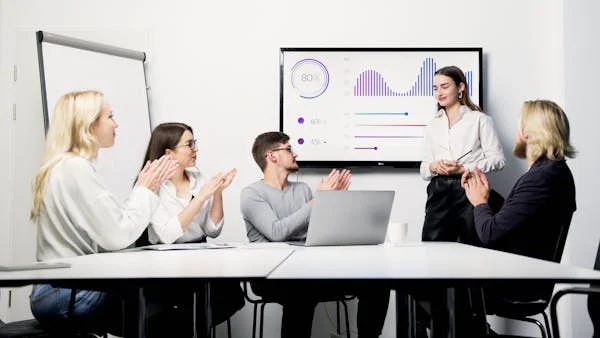
In the evolving landscape of data analysis, selecting the right tools is crucial for transforming raw data into actionable insights. With numerous options available, understanding the strengths and features of top data analysis tools can help you make an informed decision. Here’s a comprehensive comparison of the best data analysis tools for 2024, focusing on their capabilities to enhance your data analysis processes.
1. Microsoft Power BI
Overview: Microsoft Power BI stands out for its integration with Microsoft products and robust visualization capabilities. It is designed to simplify the analysis of data with user-friendly features and extensive support.
Key Features:
- Integration: Seamlessly connects with Microsoft Excel, Azure, and other Microsoft services.
- Visualization: Offers customizable dashboards and interactive reports.
- AI Insights: Includes AI-driven analytics to uncover hidden trends.
Pros: Intuitive interface, excellent for users within the Microsoft ecosystem, cost-effective options.
Cons: Limited advanced features compared to some competitors.
Image Suggestion: Screenshot of Power BI dashboard showcasing a sample interactive report.
2. Tableau
Overview: Tableau is renowned for its powerful data visualization and business intelligence features, making it ideal for detailed data analysis.
Key Features:
- Advanced Visualization: Delivers rich, interactive visualizations and dashboards.
- Real-Time Data: Enables real-time data updates and analytics.
- Data Connectivity: Connects with numerous data sources.
Pros: Highly versatile, robust for complex data visualization.
Cons: Higher cost, steeper learning curve for new users.
Image Suggestion: Example of a Tableau dashboard with various types of visualizations.
3. Google Data Studio
Overview: Google Data Studio offers a free, user-friendly platform for creating and sharing reports and dashboards, especially beneficial for users already engaged with Google’s suite of products.
Key Features:
- Integration: Integrates well with Google Analytics, Google Sheets, and other Google services.
- Collaboration: Supports real-time collaboration and sharing.
- Customizable Reports: Provides various customizable templates.
Pros: Free, easy to use, great for teams using Google tools.
Cons: Limited advanced analytics features compared to paid tools.
Image Suggestion: Screenshot of a Google Data Studio report with collaborative features highlighted.
4. Qlik Sense
Overview: Qlik Sense is known for its associative data model, which enables intuitive data exploration and discovery, enhanced by AI-driven insights.
Key Features:
- Associative Model: Facilitates easy and flexible data exploration.
- AI Insights: Provides AI-driven analytics and recommendations.
- Self-Service: Allows users to create and customize their own reports.
Pros: Flexible and powerful, ideal for in-depth data discovery.
Cons: Can be complex to set up initially.
Image Suggestion: Visual of Qlik Sense’s associative data model in action.
5. Looker
Overview: Looker, now part of Google Cloud, excels in data modeling and real-time analytics, offering strong SQL integration and powerful data exploration tools.
Key Features:
- Data Modeling: Advanced tools for data modeling and exploration.
- Real-Time Analytics: Provides real-time data access and analysis.
- SQL Integration: Seamlessly integrates with SQL databases.
Pros: Robust data modeling, excellent for real-time analytics.
Cons: Requires SQL knowledge, potentially high cost.
Image Suggestion: Looker’s data modeling interface and example real-time analytics.
6. SAS
Overview: SAS is a leading tool in advanced analytics, offering extensive features for statistical analysis, predictive modeling, and data management.
Key Features:
- Predictive Analytics: Advanced tools for forecasting and predictive modeling.
- Data Management: Comprehensive data management capabilities.
- Industry Solutions: Tailored analytics solutions for various industries.
Pros: Powerful for complex analytics and large datasets.
Cons: High cost, steep learning curve.
Image Suggestion: Screenshot of SAS’s predictive analytics tools.
7. Alteryx
Overview: Alteryx focuses on data preparation and workflow automation, making it easier to blend and analyze data efficiently.
Key Features:
- Data Blending: Streamlines data preparation and blending processes.
- Automation: Automates repetitive tasks and workflows.
- Integration: Connects with major visualization tools like Tableau and Power BI.
Pros: Efficient data preparation, strong automation features.
Cons: May require additional investment in visualization tools.
Image Suggestion: Alteryx’s workflow automation and data blending interface.
8. KNIME
Overview: KNIME is an open-source platform known for its flexible data mining and machine learning capabilities, supporting a wide range of data analysis tasks.
Key Features:
- Workflow Design: Visual drag-and-drop interface for building workflows.
- Machine Learning: Tools for machine learning and predictive analytics.
- Integration: Supports diverse data sources and analytical tools.
Pros: Free, highly customizable, suitable for complex data science projects.
Cons: Can be challenging to configure and use.
Image Suggestion: KNIME’s workflow design interface and machine learning features.
9. RapidMiner
Overview: RapidMiner offers a comprehensive platform for data science with both no-code and code-based options for analytics and machine learning.
Key Features:
- No-Code Options: User-friendly for non-technical users.
- Machine Learning: Tools for automated machine learning and predictive analytics.
- Data Preparation: Extensive features for data cleaning and preparation.
Pros: Versatile, suitable for both technical and non-technical users.
Cons: Can be costly, with a potentially steep learning curve.
Image Suggestion: RapidMiner’s no-code interface and machine learning capabilities.
10. IBM Cognos Analytics
Overview: IBM Cognos provides a comprehensive business intelligence solution with AI-driven insights and extensive reporting features.
Key Features:
- AI Insights: Enhances data exploration with AI-powered analytics.
- Reporting: Advanced reporting and dashboard capabilities.
- Natural Language Querying: Allows querying data using natural language.
Pros: Scalable, strong AI and reporting features.
Cons: High cost, more complex implementation.
Image Suggestion: IBM Cognos’s AI-driven analytics and reporting features.
Conclusion
Choosing the right data analysis tool can significantly impact how effectively you harness your data’s potential. Each tool reviewed here offers unique features and benefits, from advanced visualization and real-time analytics to robust data preparation and machine learning capabilities. By evaluating these options based on your specific needs, you can select the best tool to drive insights and enhance decision-making in 2024.
Unlocking Insights: The Best Tools for Data Analysis Reviewed
In today’s data-driven world, unlocking valuable insights requires powerful data analysis tools. With a multitude of options available, choosing the best tool can enhance your ability to interpret data, make informed decisions, and drive business success. In this review, we’ll explore some of the best tools for data analysis in 2024, highlighting their features, benefits, and unique strengths.
1. Microsoft Power BI
Overview: Microsoft Power BI is a leading tool for data analysis, known for its seamless integration with Microsoft products and its robust visualization capabilities.
Key Features:
- Integration: Connects effortlessly with Microsoft Excel, Azure, and other Microsoft services, allowing for a smooth workflow.
- Visualization: Offers customizable dashboards and interactive reports that make data easy to interpret.
- AI Insights: Provides AI-driven analytics to uncover trends and patterns in your data.
Pros: User-friendly, especially for those familiar with Microsoft products; cost-effective with various pricing options.
Cons: May lack some advanced features found in specialized tools.
Image Suggestion: Screenshot of a Power BI dashboard showcasing interactive data visualizations.
2. Tableau
Overview: Tableau excels in data visualization and business intelligence, offering powerful tools for analyzing and presenting complex data.
Key Features:
- Advanced Visualization: Known for its rich and interactive visualizations that facilitate in-depth data analysis.
- Real-Time Data: Enables real-time data updates, ensuring that your analyses reflect the most current information.
- Data Connectivity: Connects with a wide range of data sources for comprehensive analysis.
Pros: Highly versatile with strong visualization capabilities; suitable for complex data analysis tasks.
Cons: Higher cost; can be challenging for new users to master.
Image Suggestion: Example of a Tableau visualization, including various chart types and interactive elements.
3. Google Data Studio
Overview: Google Data Studio provides a free, accessible platform for creating and sharing data reports and dashboards, ideal for users within the Google ecosystem.
Key Features:
- Integration: Integrates smoothly with Google Analytics, Google Sheets, and other Google services, streamlining data analysis.
- Collaboration: Supports real-time collaboration and sharing of reports and dashboards.
- Customizable Reports: Offers a variety of customizable templates for different reporting needs.
Pros: Free and easy to use; excellent for users already using Google products.
Cons: Limited advanced features compared to premium tools.
Image Suggestion: Google Data Studio report with collaborative features and customizable templates highlighted.
4. Qlik Sense
Overview: Qlik Sense is known for its associative data model and AI-driven insights, providing a powerful platform for data exploration and discovery.
Key Features:
- Associative Model: Facilitates intuitive and flexible data exploration, allowing users to easily discover insights.
- AI Insights: Utilizes AI to provide data-driven recommendations and insights.
- Self-Service: Empowers users to create and customize their own reports and dashboards.
Pros: Highly flexible and powerful for data discovery; great for users needing advanced analysis.
Cons: Can be complex to set up; initial learning curve may be steep.
Image Suggestion: Visual of Qlik Sense’s associative data model in use, showcasing interactive data exploration.
5. Looker
Overview: Looker, now part of Google Cloud, is known for its robust data modeling and real-time analytics capabilities, making it a powerful tool for data analysis.
Key Features:
- Data Modeling: Provides advanced tools for data modeling, enabling detailed and accurate analysis.
- Real-Time Analytics: Offers real-time data access, ensuring that your insights are up-to-date.
- SQL Integration: Integrates seamlessly with SQL databases for enhanced data manipulation.
Pros: Strong data modeling and real-time analytics capabilities; suitable for complex analytical tasks.
Cons: Requires SQL knowledge; can be expensive.
Image Suggestion: Looker’s data modeling interface and real-time analytics in action.
6. SAS
Overview: SAS is a leader in advanced analytics, offering a suite of tools for statistical analysis, predictive modeling, and data management.
Key Features:
- Predictive Analytics: Provides tools for advanced forecasting and predictive modeling.
- Data Management: Comprehensive capabilities for managing and analyzing large datasets.
- Industry Solutions: Tailored analytics solutions for various industries.
Pros: Powerful for complex and large-scale data analysis; well-suited for industries with specialized needs.
Cons: High cost; steep learning curve for new users.
Image Suggestion: SAS’s predictive analytics tools and data management features.
7. Alteryx
Overview: Alteryx focuses on data preparation and workflow automation, helping users blend and analyze data efficiently.
Key Features:
- Data Blending: Simplifies the process of combining data from various sources.
- Automation: Automates repetitive data tasks and workflows, improving efficiency.
- Integration: Works well with visualization tools like Tableau and Power BI.
Pros: Efficient for data preparation and workflow automation; integrates well with other tools.
Cons: Additional investment needed for visualization tools.
Image Suggestion: Alteryx’s data blending and workflow automation interface.
8. KNIME
Overview: KNIME is an open-source platform known for its flexibility in data mining and machine learning.
Key Features:
- Workflow Design: Features a visual drag-and-drop interface for designing data workflows.
- Machine Learning: Offers tools for building and deploying machine learning models.
- Integration: Supports a wide range of data sources and analytical tools.
Pros: Free and customizable; suitable for complex data science projects.
Cons: Configuration can be challenging; may require technical expertise.
Image Suggestion: KNIME’s workflow design and machine learning capabilities.
9. RapidMiner
Overview: RapidMiner provides a comprehensive platform for data science, offering both no-code and code-based options for analytics.
Key Features:
- No-Code Options: User-friendly interface for non-technical users.
- Machine Learning: Includes tools for automated machine learning and predictive analytics.
- Data Preparation: Extensive features for data cleaning and preparation.
Pros: Versatile for both technical and non-technical users; strong data preparation features.
Cons: Can be costly; initial learning curve.
Image Suggestion: RapidMiner’s no-code interface and machine learning tools.
10. IBM Cognos Analytics
Overview: IBM Cognos Analytics offers a comprehensive business intelligence solution with AI-driven insights and extensive reporting capabilities.
Key Features:
- AI Insights: Enhances data exploration with AI-powered analytics.
- Reporting: Advanced reporting and dashboard features.
- Natural Language Querying: Allows users to query data using natural language.
Pros: Scalable and powerful; strong AI and reporting features.
Cons: Higher cost; more complex implementation.
Image Suggestion: IBM Cognos’s reporting features and AI-driven analytics.
Conclusion
Unlocking insights from your data requires powerful and effective tools. Each of the reviewed data analysis tools offers unique features that cater to various needs, from advanced visualization and real-time analytics to efficient data preparation and machine learning. By selecting the right tool based on your specific requirements, you can maximize the value of your data and make informed, data-driven decisions.
Image Suggestion: Comparative table or chart summarizing key features and benefits of each tool for a visual summary.
Choosing the Right Tool for Analysis of Data: A 2024 Guide
Selecting the right tool for the analysis of data is crucial for leveraging insights effectively and driving business success. With numerous data analysis tools available in 2024, making the right choice requires understanding your needs, evaluating key features, and comparing various options. This guide will help you navigate the decision-making process to choose the best tool for your data analysis needs.
1. Assess Your Needs
Understand Your Requirements: Begin by identifying your specific data analysis needs. Consider factors such as the type of data you work with, the complexity of analysis required, and the level of detail you need in your reports.
Determine Key Features: Think about the essential features you need, such as advanced analytics, data visualization, integration capabilities, and user-friendliness. This will help narrow down your options to tools that meet your core requirements.
Image Suggestion: Diagram or checklist highlighting key questions to consider when assessing data analysis needs.
2. Evaluate Core Features
Data Integration: Ensure the tool can integrate with your existing data sources and platforms. Compatibility with databases, spreadsheets, and cloud services is essential for seamless data import and export.
Analysis Capabilities: Look for tools that offer the types of analysis you need, such as descriptive statistics, predictive modeling, or advanced machine learning. Some tools provide more specialized features, so choose one that aligns with your analytical goals.
Visualization Options: Effective data visualization is crucial for interpreting data insights. Evaluate the tool’s capabilities for creating interactive and customizable dashboards, charts, and graphs.
Image Suggestion: Screenshots or examples of data integration and visualization features from different tools.
3. Consider Usability
User Interface: The tool should have an intuitive and user-friendly interface. Consider how easy it is to navigate, create reports, and analyze data without extensive training.
Learning Curve: Assess how long it will take for your team to become proficient with the tool. Some tools are designed for ease of use, while others may have a steeper learning curve.
Support and Training: Check if the tool offers adequate support and training resources, such as tutorials, webinars, and customer support channels.
Image Suggestion: Comparative chart of user interface designs and learning curves of popular data analysis tools.
4. Review Performance and Scalability
Handling Large Datasets: Ensure the tool can manage large volumes of data efficiently without performance issues. Scalability is important as your data needs grow.
Speed and Efficiency: Evaluate the tool’s speed in processing and analyzing data. Tools that offer faster performance can enhance productivity and decision-making.
Scalability: Consider whether the tool can scale with your business. It should handle increased data volumes and complexity as your needs evolve.
Image Suggestion: Performance comparison graph showing processing speeds and scalability options of different tools.
5. Compare Pricing and Value
Pricing Models: Understand the tool’s pricing structure, whether it’s subscription-based, one-time purchase, or tiered pricing. Compare these models with your budget and expected usage.
Cost vs. Value: Evaluate the value you get from the tool in relation to its cost. Consider factors such as features, ease of use, and support when determining if it offers good value for your investment.
Trial and Free Versions: Many tools offer free trials or demo versions. Take advantage of these to test the tool’s capabilities and fit for your needs before committing to a purchase.
Image Suggestion: Table comparing pricing models and features of different data analysis tools.
6. Assess Security and Compliance
Data Security: Ensure the tool provides robust security features, such as encryption and access controls, to protect your data.
Compliance: Verify that the tool complies with industry regulations and standards, especially if you handle sensitive or regulated data.
Privacy Measures: Look for features that support data privacy and confidentiality, such as user permissions and audit trails.
Image Suggestion: Visual representation of security features and compliance certifications of various tools.
7. Consider Customer Reviews and Case Studies
User Feedback: Read reviews and testimonials from other users to gauge their experiences with the tool. Look for feedback on performance, support, and overall satisfaction.
Case Studies: Review case studies or success stories to see how other businesses have used the tool and the results they achieved. This can provide insights into its effectiveness in real-world scenarios.
Image Suggestion: Summary of user reviews and case study highlights for different data analysis tools.
Conclusion
Choosing the right tool for the analysis of data in 2024 involves carefully evaluating your needs, comparing features, and considering factors such as usability, performance, pricing, and security. By following this guide, you can make an informed decision and select a data analysis tool that will help you unlock valuable insights and drive better business outcomes.
Image Suggestion: Final comparison chart or decision matrix summarizing key features, benefits, and considerations for choosing the best data analysis tool.
Data Analysis Tools Compared: Features, Pricing, and Performance
When choosing the right data analysis tool, it’s essential to compare features, pricing, and performance to ensure you select a solution that meets your needs effectively. This comparison of leading data analysis tools will provide a clear view of what each tool offers, helping you make an informed decision.
1. Microsoft Power BI
Features:
- Integration: Seamlessly integrates with Microsoft Excel, Azure, and other Microsoft products.
- Visualization: Offers customizable dashboards and interactive reports.
- AI Insights: Includes AI-driven analytics to uncover trends and patterns.
Pricing:
- Free Version: Limited features with basic functionalities.
- Pro Version: Approximately $9.99 per user per month, offering enhanced features and collaboration tools.
- Premium Version: Pricing varies based on capacity and advanced features.
Performance:
- Speed: Efficient for real-time data updates and large datasets.
- Scalability: Scales well with organizational needs, though higher-tier options are available for larger enterprises.
Image Suggestion: Pricing table showing different tiers and features of Microsoft Power BI.
2. Tableau
Features:
- Advanced Visualization: Known for its rich, interactive visualizations and dashboards.
- Real-Time Data: Allows real-time data analysis and updates.
- Data Connectivity: Connects with a wide range of data sources.
Pricing:
- Individual License: Starts at around $70 per user per month.
- Team and Enterprise Licenses: Higher pricing for additional features and support, with custom quotes based on requirements.
Performance:
- Speed: Fast data processing and visualization capabilities.
- Scalability: Suitable for both small and large-scale data analysis, with options for enterprise-level deployments.
Image Suggestion: Example of Tableau visualizations with performance metrics.
3. Google Data Studio
Features:
- Integration: Works seamlessly with Google Analytics, Google Sheets, and other Google services.
- Collaboration: Supports real-time collaboration and sharing.
- Customizable Reports: Offers various customizable templates for reports.
Pricing:
- Free: No cost for the basic version with essential features.
Performance:
- Speed: Generally fast for standard data visualization and reporting tasks.
- Scalability: Suitable for most small to medium-sized data projects; may be less effective for very large datasets.
Image Suggestion: Screenshot of a Google Data Studio report highlighting collaborative features.
4. Qlik Sense
Features:
- Associative Model: Facilitates intuitive data exploration and discovery.
- AI Insights: Provides AI-driven recommendations and insights.
- Self-Service: Allows users to create and customize reports and dashboards.
Pricing:
- Business License: Around $30 per user per month.
- Enterprise License: Custom pricing based on the scale and specific needs.
Performance:
- Speed: Highly efficient for complex data analysis and interactive exploration.
- Scalability: Effective for both small and large datasets, with strong enterprise capabilities.
Image Suggestion: Visual of Qlik Sense’s associative data model and interactive exploration.
5. Looker
Features:
- Data Modeling: Advanced tools for data modeling and exploration.
- Real-Time Analytics: Provides up-to-date data access and analysis.
- SQL Integration: Seamless integration with SQL databases.
Pricing:
- Custom Pricing: Based on specific requirements and scale, with no fixed pricing available online.
Performance:
- Speed: Strong real-time analytics capabilities.
- Scalability: Scales well with complex data needs and large enterprises.
Image Suggestion: Looker’s data modeling and real-time analytics interface.
6. SAS
Features:
- Predictive Analytics: Advanced tools for statistical analysis and predictive modeling.
- Data Management: Comprehensive data management capabilities.
- Industry Solutions: Tailored analytics solutions for various industries.
Pricing:
- Custom Pricing: Typically higher cost with pricing based on specific needs and enterprise scale.
Performance:
- Speed: Powerful for large-scale data analysis and complex predictive modeling.
- Scalability: Excellent for enterprise-level data analysis with extensive scalability options.
Image Suggestion: Screenshot showcasing SAS’s predictive analytics and data management features.
7. Alteryx
Features:
- Data Blending: Simplifies combining data from various sources.
- Automation: Automates repetitive tasks and workflows.
- Integration: Works with major visualization tools like Tableau and Power BI.
Pricing:
- Designer License: Starts at approximately $5,195 per user per year.
- Server and Analytics Hub: Custom pricing for larger deployments.
Performance:
- Speed: Efficient for data preparation and workflow automation.
- Scalability: Suitable for various sizes of data projects, with extensive automation capabilities.
Image Suggestion: Alteryx’s data blending and workflow automation features.
8. KNIME
Features:
- Workflow Design: Visual drag-and-drop interface for creating workflows.
- Machine Learning: Tools for machine learning and data mining.
- Integration: Supports various data sources and analytical tools.
Pricing:
- Open Source: Free for the basic version with community support.
- Commercial Extensions: Pricing varies for enterprise features and support.
Performance:
- Speed: Good for complex workflows and large datasets.
- Scalability: Highly flexible and suitable for extensive data science projects.
Image Suggestion: KNIME’s visual workflow design and machine learning capabilities.
9. RapidMiner
Features:
- No-Code Options: User-friendly interface for non-technical users.
- Machine Learning: Includes automated machine learning and predictive analytics tools.
- Data Preparation: Comprehensive data cleaning and preparation features.
Pricing:
- Free Version: Basic functionalities with limitations.
- Paid Versions: Start at around $2,500 per user per year, with higher tiers available.
Performance:
- Speed: Effective for both data preparation and analysis tasks.
- Scalability: Versatile, with options for both small and large-scale data analysis.
Image Suggestion: RapidMiner’s no-code interface and machine learning tools.
10. IBM Cognos Analytics
Features:
- AI Insights: Enhances data exploration with AI-driven analytics.
- Reporting: Advanced reporting and dashboard capabilities.
- Natural Language Querying: Allows querying using natural language.
Pricing:
- Basic Plans: Around $10 per user per month.
- Enterprise Plans: Custom pricing based on organizational needs and scale.
Performance:
- Speed: Strong performance in reporting and AI-driven insights.
- Scalability: Well-suited for large enterprises with extensive data needs.
Image Suggestion: IBM Cognos’s reporting features and natural language querying interface.
Conclusion
Choosing the right data analysis tool involves comparing features, pricing, and performance to find a solution that meets your specific needs. Each tool reviewed here offers unique capabilities, from advanced visualizations and real-time analytics to data blending and machine learning. By evaluating these aspects, you can select a tool that will help you unlock valuable insights and enhance your data-driven decision-making processes.
Image Suggestion: Comparative summary chart or table highlighting key features, pricing, and performance metrics of each data analysis tool.
The Ultimate Guide to Data Analysis Tools: What You Need to Know
In the era of big data, choosing the right data analysis tools is essential for transforming raw data into actionable insights. Whether you’re a business analyst, data scientist, or just getting started with data analysis, understanding the features, capabilities, and benefits of various tools is crucial. This ultimate guide will walk you through everything you need to know about data analysis tools to help you make an informed choice.
1. Understanding Data Analysis Tools
What Are Data Analysis Tools?: Data analysis tools are software applications designed to process, analyze, and visualize data. They help users identify patterns, trends, and insights from large datasets, making it easier to make data-driven decisions.
Types of Data Analysis Tools:
- Statistical Tools: Focus on statistical analysis and modeling (e.g., SAS, R).
- Visualization Tools: Specialize in creating charts, graphs, and dashboards (e.g., Tableau, Microsoft Power BI).
- Data Preparation Tools: Handle data cleaning, blending, and transformation (e.g., Alteryx, KNIME).
Image Suggestion: Diagram or infographic illustrating the different types of data analysis tools and their primary functions.
2. Key Features to Look For
Integration Capabilities: The ability to connect with various data sources, such as databases, spreadsheets, and cloud services, is essential for comprehensive analysis.
Advanced Analytics: Look for tools that offer advanced analytics capabilities, such as predictive modeling, machine learning, and statistical analysis.
Visualization Options: Effective visualization is crucial for interpreting data. Choose tools that provide customizable dashboards, interactive charts, and easy-to-read graphs.
User Interface: A user-friendly interface is important for efficiency. The tool should be intuitive and easy to navigate, with minimal training required.
Scalability: Ensure the tool can handle your current data needs and scale as your data grows. This is particularly important for large enterprises and data-heavy projects.
Image Suggestion: Features comparison chart showing key features of various data analysis tools.
3. Evaluating Pricing Models
Free vs. Paid Versions: Many tools offer free versions with limited features and paid versions with enhanced capabilities. Evaluate which features are critical for your needs and whether the cost justifies the benefits.
Subscription Models: Most modern tools use a subscription-based pricing model, which can be monthly or annual. Compare the costs and benefits of different subscription tiers.
Enterprise Pricing: For large organizations, custom pricing may be available. This can include additional support, advanced features, and scalability options.
Image Suggestion: Pricing comparison table with different subscription models and features included in each tier.
4. Performance Considerations
Speed: The tool should efficiently handle data processing and analysis, especially if you’re dealing with large datasets.
Reliability: Ensure the tool performs consistently without frequent crashes or slowdowns. Check for user reviews and performance benchmarks.
Support and Updates: Regular updates and reliable customer support are important for maintaining tool performance and addressing any issues that arise.
Image Suggestion: Performance comparison graph showing speed and reliability metrics of various data analysis tools.
5. Security and Compliance
Data Security: Choose tools that offer robust security features, such as data encryption, user access controls, and secure data storage.
Compliance: Ensure the tool complies with industry regulations and standards, particularly if you handle sensitive or regulated data.
Privacy Measures: Look for tools that provide privacy controls and data protection features to safeguard sensitive information.
Image Suggestion: Security and compliance checklist or infographic highlighting key security features.
6. Making the Right Choice
Match Features to Needs: Align the tool’s features with your specific data analysis requirements. For example, if you need advanced statistical analysis, focus on tools with strong statistical capabilities.
Trial and Testing: Many tools offer free trials or demo versions. Take advantage of these to test the tool’s functionality and suitability for your needs before making a purchase.
Consider User Feedback: Review user testimonials and case studies to understand how others have benefited from the tool and any potential limitations.
Image Suggestion: Flowchart or decision matrix to help guide the selection process based on specific needs and features.
Conclusion
Choosing the right data analysis tools involves understanding their features, evaluating pricing models, considering performance, and ensuring security and compliance. By following this ultimate guide, you’ll be equipped with the knowledge needed to select a tool that best fits your needs, enhances your data analysis capabilities, and supports your data-driven decision-making process.
Image Suggestion: Summary chart or infographic consolidating key points from the guide, such as features, pricing, and performance considerations for various data analysis tools.